Denoise image using Kornia and Ivy’s Transpiler#
Introduction#
This tutorial is adapted from Kornia’s official tutorial. We demonstrate how to denoise an image using the differentiable total_variation loss, leveraging Ivy’s Transpiler to run these augmentations in TensorFlow using ivy.transpile
.
Original Tutorial Credits#
The original tutorial and code are courtesy of Kornia. This notebook serves as an adapted version, showcasing the integration with Ivy’s Transpiler for TensorFlow compatibility.
Installation and Setup#
[1]:
%%capture
!pip install kornia
!pip install kornia-rs
!pip install matplotlib
!pip install -q ivy
!rm -rf ivy_transpiled_outputs/
[2]:
import kornia as K
import matplotlib.pyplot as plt
import torch
import torchvision
import ivy
import tensorflow as tf
/usr/local/lib/python3.10/dist-packages/kornia/feature/lightglue.py:44: FutureWarning: `torch.cuda.amp.custom_fwd(args...)` is deprecated. Please use `torch.amp.custom_fwd(args..., device_type='cuda')` instead.
@torch.cuda.amp.custom_fwd(cast_inputs=torch.float32)
This line lazily transpiles everything in the kornia api to TensorFlow
, and creates a new module for this transpiled version of kornia. Because the transpilation happens lazily, no function or class will be transpiled until it’s actually called.
[3]:
tf_kornia = ivy.transpile(K, source='torch', target="tensorflow")
[4]:
import io
import requests
def download_image(url: str, filename: str = "") -> str:
filename = url.split("/")[-1] if len(filename) == 0 else filename
# Download
bytesio = io.BytesIO(requests.get(url).content)
# Save file
with open(filename, "wb") as outfile:
outfile.write(bytesio.getbuffer())
return filename
url = "https://github.com/kornia/data/raw/main/doraemon.png"
download_image(url)
[4]:
'doraemon.png'
Defining imshow
function#
[5]:
%%writefile imshow.py
import kornia as K
import matplotlib.pyplot as plt
import torch
import torchvision
def imshow(input: torch.Tensor):
out = torchvision.utils.make_grid(input, nrow=2, padding=5)
out_np = K.utils.tensor_to_image(out)
plt.imshow(out_np)
plt.axis("off")
plt.show()
Writing imshow.py
Transpiling imshow
Function to TensorFlow#
[6]:
from imshow import imshow
transpiled_imshow = ivy.transpile(imshow, source='torch', target='tensorflow')
Transpilation of imshow complete.
loading an visualizing the image.#
This cell triggers the transpilation of kornia.io.load_image
from PyTorch to TensorFlow. Due to Ivy’s lazy transpilation, the actual compilation occurs only when the function is called.
[7]:
# read the image with kornia and add a random noise to it
img = tf_kornia.io.load_image("doraemon.png", tf_kornia.io.ImageLoadType.RGB32) # CxHxW
noise = tf.random.normal(shape=tf.shape(img), mean=0.0, stddev=0.1)
# Add noise to the image and clamp values between 0 and 1
noisy_image_tf = tf.clip_by_value(img + noise, 0.0, 1.0)
transpiled_imshow(noisy_image_tf)
Transpilation of load_image complete.
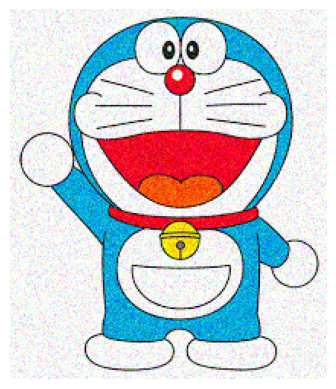
Defining Total Variation Denoising Network#
[8]:
%%writefile TVDenoise.py
import torch
import kornia as K
class TVDenoise(torch.nn.Module):
def __init__(self, noisy_image):
super().__init__()
self.l2_term = torch.nn.MSELoss(reduction="mean")
self.regularization_term = K.losses.TotalVariation()
# create the variable which will be optimized to produce the noise free image
self.clean_image = torch.nn.Parameter(data=noisy_image.clone(), requires_grad=True)
def forward(self, noisy_image):
return self.l2_term(self.clean_image, noisy_image) + 0.0001 * self.regularization_term(self.clean_image)
def get_clean_image(self):
return self.clean_image
Writing TVDenoise.py
Transpiling TVDenoise
Network to TensorFlow#
[9]:
from TVDenoise import TVDenoise
transpiledTVDenoise = ivy.transpile(TVDenoise, source="torch", target="tensorflow")
Transpilation of TVDenoise complete.
Running Optimization Loop#
[10]:
tv_denoiser = transpiledTVDenoise(noisy_image_tf)
optimizer = tf.keras.optimizers.SGD(learning_rate=0.1, momentum=0.9)
num_iters = 500
for i in range(num_iters):
with tf.GradientTape() as tape:
loss = tf.reduce_sum(tv_denoiser(noisy_image_tf))
# Every 50 iterations, print the loss
if i % 50 == 0:
print(f"Loss in iteration {i} of {num_iters}: {loss.numpy():.3f}")
# Compute gradients and apply optimization step
gradients = tape.gradient(loss, tv_denoiser.trainable_variables)
optimizer.apply_gradients(zip(gradients, tv_denoiser.trainable_variables))
Loss in iteration 0 of 500: 3.075
Loss in iteration 50 of 500: 2.718
Loss in iteration 100 of 500: 2.352
Loss in iteration 150 of 500: 2.057
Loss in iteration 200 of 500: 1.822
Loss in iteration 250 of 500: 1.637
Loss in iteration 300 of 500: 1.493
Loss in iteration 350 of 500: 1.381
Loss in iteration 400 of 500: 1.294
Loss in iteration 450 of 500: 1.227
Visualizing Results#
[11]:
# convert back to numpy
img_clean = tf_kornia.utils.tensor_to_image(tv_denoiser.get_clean_image())
# Create the plot
fig, axs = plt.subplots(1, 2, figsize=(16, 10))
axs = axs.ravel()
axs[0].axis("off")
axs[0].set_title("Noisy image")
axs[0].imshow(tf_kornia.tensor_to_image(noisy_image_tf))
axs[1].axis("off")
axs[1].set_title("Cleaned image")
axs[1].imshow(img_clean)
plt.show()
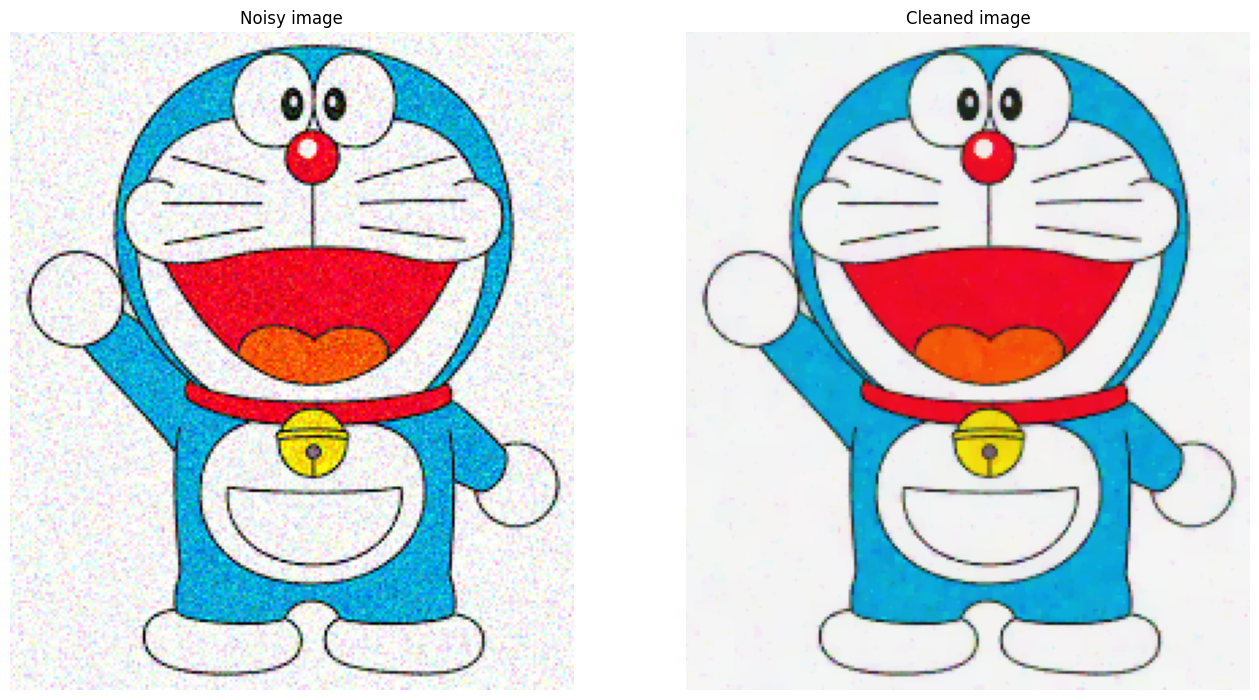
Conclusion#
In this tutorial, we demonstrated how to leverage Ivy’s Transpiler to run Kornia’s total variation denoising algorithm in TensorFlow.
What’s Next?#
Want to explore more? Dive into our documentation for in-depth guides, tutorials, and API references. Your feedback is invaluable – join our Discord to share your thoughts and suggestions.